The mathematical statistics group does research both in statistical methodology and the application of such methodology to real life problems. The group mainly concentrates on the following research fields:
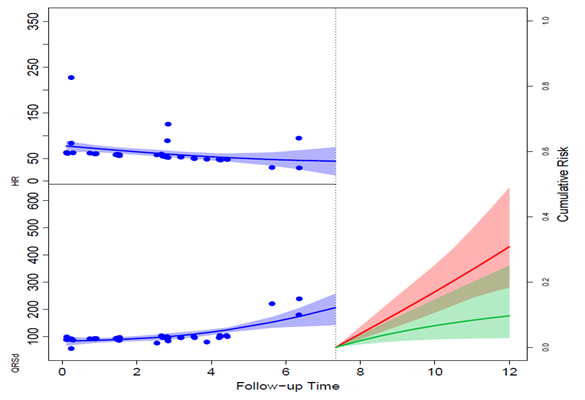
Analysis of time to event data
Contact person: Jan Terje Kvaløy
Time to event data is important in many fields like medicine, engineering and social science, and this type of data require specific statistical methods due to issues like incomplete observations, skew distributions, competing risks etc.
The research activities in time to event data spans from applied work with medical and engineering data to methodological work. Some areas of interest for methodological research are flexible non-parametric modelling, residual analysis and analysis of trends in recurrent event data.
On the applied side we work e.g. with relative survival analysis of cancer registry data, multi-state modelling of emergency medicine data and exponential regression of nucleation time data.
Statistical processes control
Contact person: Jan Terje Kvaløy
Statistical process control is a set of techniques for monitoring and controlling stochastic processes over time with applications in industry, medicine, environmental monitoring etc. A key method is control charts for monitoring of processes.
Current research interests are into methods for monitoring time to event data med methodsfor model choice when fitting risk adjusted control charts. We also work on applications to medical data.
Computational Statistics
Contact person: Tore Selland Kleppe
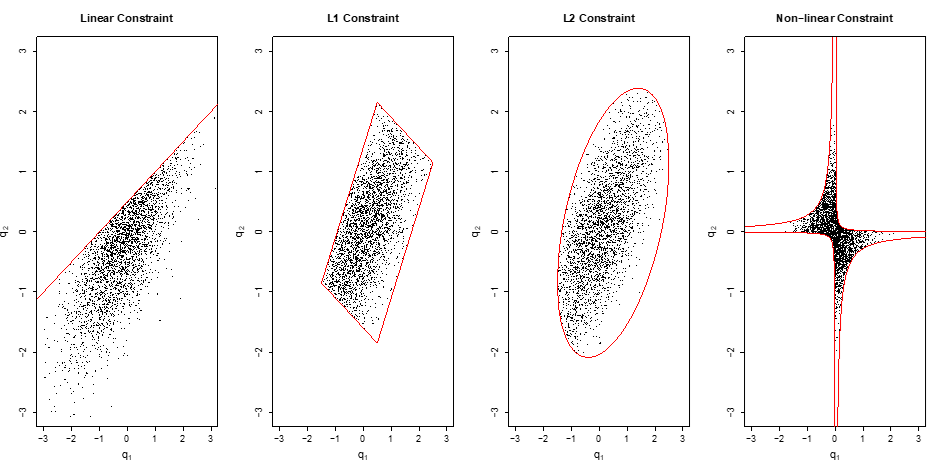
In many situations, non-linear statistical models are needed for an appropriate modeling of the process which is generating data. In such cases, computational statistical methods are typically required.
The end of such methods is typically to numerically compute integrals (as opposed to finding analytic expressions) over high-dimensional spaces, which are needed for analyzing non-linear statistical models and fitting such models to data.
Currently, the research focus in computational statistics is mainly centered on Hamiltonian Monte Carlo (HMC) algorithms for Bayesian hierarchical models. HMC algorithms produces random numbers with a given distribution by simulating Hamiltonian dynamical systems.
The ongoing work involves international collaborators and draws on insights from many different fields, including differential geometry and physics, numerical methods (symplectic integrators, sparse numerical linear algebra, automatic differentiation), numerical optimization as well as Bayesian statistics.
A large part of this ongoing effort is implemented in the R-package pdmphmc, which is documented at https://torekleppe.github.io/pdmphmc.doc/
In our project “Connecting the dots: Automated inference for large scale Bayesian econometric models” (2023-2027), funded by “Finansmarkedsfondet”, we aim to further develop such methodology, with particular emphasis on methodology that may be used for building large scale econometric models.
Econometric time series analysis
Contact person: Tore Selland Kleppe
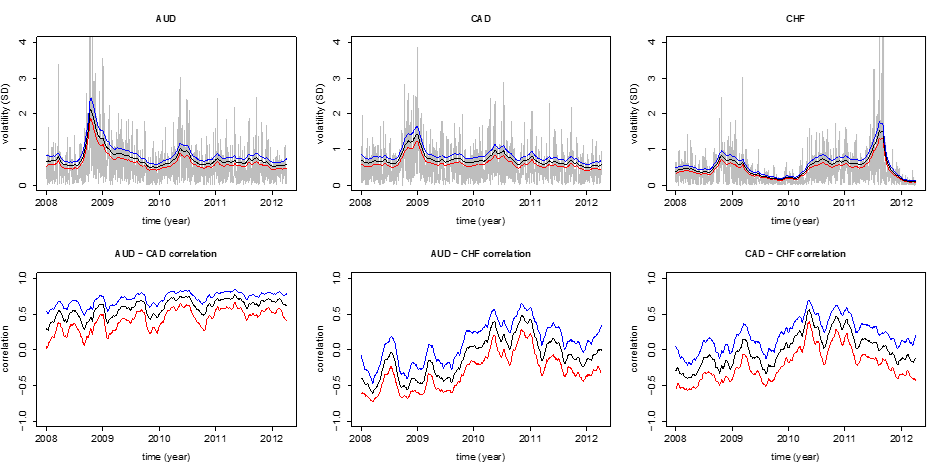
Currently, the ongoing work in econometric time series analysis centers mainly around non-linear models for commodities market (e.g. crude oil, natural gas, agricultural commodities), and in particular modeling of futures markets.
The work is done in cooperation both with the UiS industrial economics researchers, and international collaborators. We consider both reduced form models (i.e. not based on economic theory) and more theoretically founded models are considered.
In many cases, such models are cast in terms of Bayesian hierarchical models, and the numerical methods required for fitting such models to data are a large part of the research activity.
Statistical shape analysis and complex data
Contact person: Jörn Schulz
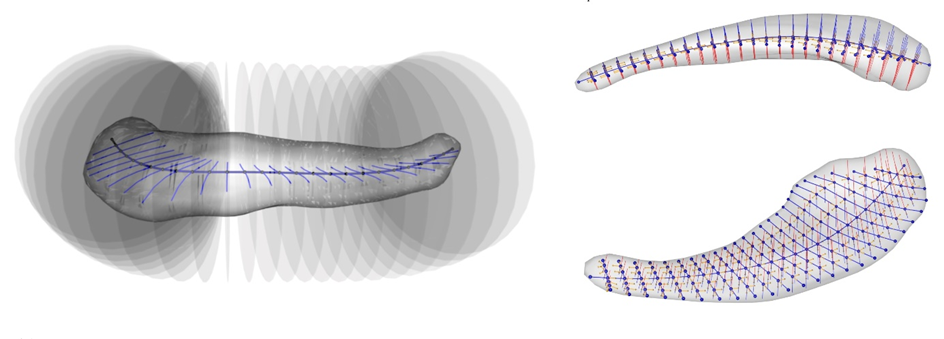
Statistical shape analysis is a multidisciplinary field involving statistics, mathematics and computer vision on complex data. One powerful approach for shape analysis of slabular objects is based on their skeletal representation that outperforms other shape models like point distribution models by capturing the interior of the objects.
We advance current shape models for example by the locally parameterized discrete skeletal representation that is invariant to rigid transformation and defines good correspondence across a population of objects. Parallel to this, we develop powerful and robust statistical methods to fully exploit the entire potential for complex data such as shape data that is living on a product on linear and non-linear data spaces. Such methods include for example a sensitive notation of a mean, hypothesis testing and how to describe the modes of variation for complex data.
Bayesian analysis of multivariate spatial and temporal data
Contact person: Arild Buland
Bayesian methodology provides a general statistical framework to integrate current knowledge and belief with new information or data to get an updated posterior model. For non-linear and ill-posed inverse problems, the Bayesian method will also have an important stabilizing effect on the predictions. Spatial and temporal models are important in many problems in earth sciences and signal analysis.
The research activity is mainly related to geological earth models and geophysical data and signals, such as analysis of seismic signal for prediction of discrete lithology and fluid types and continuous parameters such as reservoir porosity, saturation, and pressure.
Medical statistics
Contact persons: Bjørn Auestad, Jan Terje Kvaløy and Jörn Schulz
The statistics group have extensive collaborations with researchers in medical sciences, in particular at SUS but also at UiB, UiO and NTNU. In these medical research projects, we analyze a wide variety of different types of data, and we also contribute to the planning of studies.
In these projects many elements of the statistical toolbox are applied, adapted, and extended. Some of the work on medical problems is in collaboration with researchers at the Department of Electrical Engineering and Computer Science at UiS as part of the research group “BMDLab – Biomedical Data Analysis Laboratory”, and we also have some collaboration with researchers at the Faculty of Health Sciences at UiS.
Statistical modeling of meteorological processes
Contact person: Bjørn Auestad
Various kinds of meteorological data sampled at fine resolution become more and more available. There is research activity focused on exploiting such data in connection with analyses of climate changes. We work currently with Markov models for daily precipitation data.
The model is time inhomogeneous to account for seasonal effects and orders larger than one is considered. One important aspect of precipitation processes is the properties of the spell length distribution; e.g. the distribution of the number of consecutive wet/dry days.
These properties are also generally seasonally dependent. The Markov approach seems promising for such analyses. The research activity centers around developing methods based on the Markov model approach to study possible changes over time.
Group members, permanent (in alphabetical order)
Group members, non-permanent
Department of Mathematics and Physics
Department of Mathematics and Physics
Former group members
- Stein Andreas Bethuelsen (Associate Professor)
- Kjartan Kloster Osmundsen (PhD student)
- Birthe Aarekol Lindaas (PhD student)
- Berent Ånund Strømnes Lunde (PhD student)